- April 2, 2023
- Posted by: admin
- Category: Storage
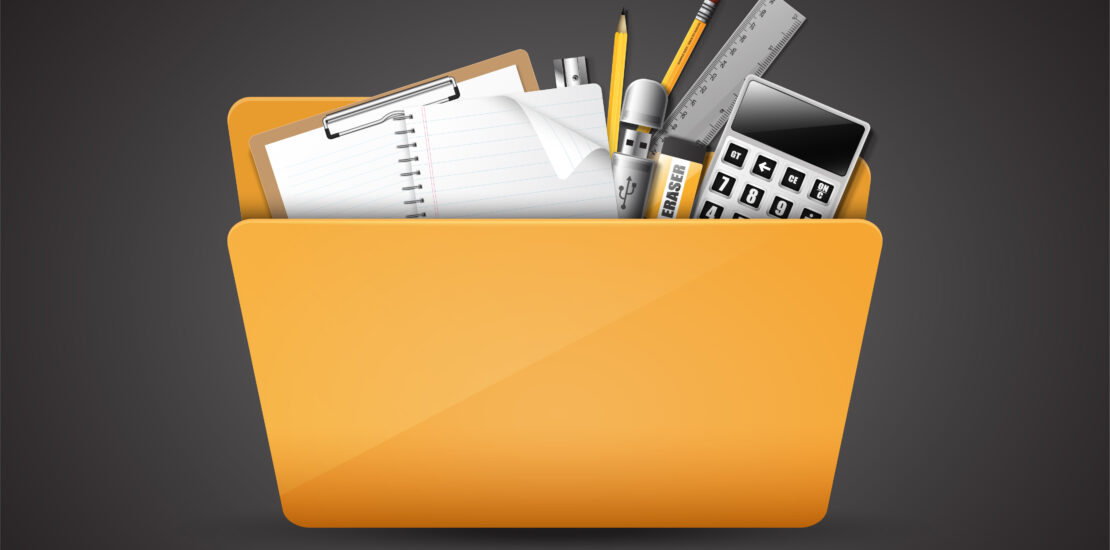
Introduction:
In today’s digital era, the exponential growth of data has brought about numerous challenges, especially in sectors such as Internet of Things (IoT), media, and artificial intelligence (AI). With IoT applications generating vast amounts of data, media companies handling large files, and AI companies working with extensive datasets comprising videos, images, and text files, the need for efficient storage management has become paramount. This article explores the problem of managing storage in these domains and highlights potential solutions to cope with the data overload.
The Rise of IoT: The proliferation of IoT devices has resulted in an explosion of data. Sensors embedded in various devices, ranging from smart homes to industrial machinery, generate a continuous stream of information. Managing and storing this colossal volume of data poses significant challenges for organizations. Traditional storage systems struggle to keep pace with the sheer volume, velocity, and variety of IoT-generated data.
Media Companies and Large Files: Media companies, including those involved in film production, streaming services, and digital advertising, face the daunting task of managing large files. High-resolution videos, uncompressed images, and audio recordings consume substantial storage space. Storing, organizing, and retrieving these files in a timely and efficient manner becomes increasingly complex as the volume and quality of media content continue to grow.
AI Companies and Massive Datasets: AI companies heavily rely on vast datasets to train machine learning models. These datasets often include a combination of textual data, images, and videos. As AI algorithms become more sophisticated, the demand for larger datasets escalates. Managing these massive datasets efficiently is crucial for AI companies to ensure accurate training, testing, and validation of their models. Moreover, the ability to store and access these datasets promptly is critical for iterative improvements and iterative machine learning processes.
Challenges in Storage Management:
The challenges associated with managing storage in these domains are multi-fold. They include:
- Scalability: The sheer volume of data generated by IoT applications, media companies, and AI firms demands scalable storage solutions. Traditional storage systems may struggle to handle the exponential growth, leading to bottlenecks and performance issues.
- Speed and Accessibility: Quick access to stored data is crucial for real-time decision-making and efficient operations. Slow retrieval times hinder productivity and can have adverse effects on critical processes.
- Data Security: With sensitive and proprietary data at stake, ensuring robust security measures becomes paramount. Protecting data from unauthorized access, accidental deletion, or cyber threats is a constant concern.
- Cost Optimization: As data storage needs grow, so does the cost. Organizations need to strike a balance between storage capacity, performance, and budgetary constraints.
Solutions and Best Practices:
To address the storage challenges posed by IoT, media, and AI domains, organizations can adopt the following solutions and best practices:
- Cloud Storage and Hybrid Solutions: Leveraging cloud storage services allows organizations to offload the burden of managing physical storage infrastructure. Cloud providers offer scalable, flexible, and cost-effective storage options, enabling organizations to handle growing data volumes seamlessly. Hybrid solutions combining on-premises storage with cloud services provide a balance between data security, accessibility, and scalability.
- Data Compression and Deduplication: Employing efficient compression algorithms and deduplication techniques can significantly reduce storage requirements. These techniques eliminate redundant data and optimize storage capacity, enabling organizations to store more data without incurring excessive costs.
- Tiered Storage: Implementing tiered storage architectures allows organizations to prioritize data based on its frequency of use, criticality, or performance requirements. Frequently accessed data can be stored on high-performance storage tiers, while less frequently accessed data can be stored on lower-cost, high-capacity tiers.
- Data Lifecycle Management: Developing comprehensive data lifecycle management strategies